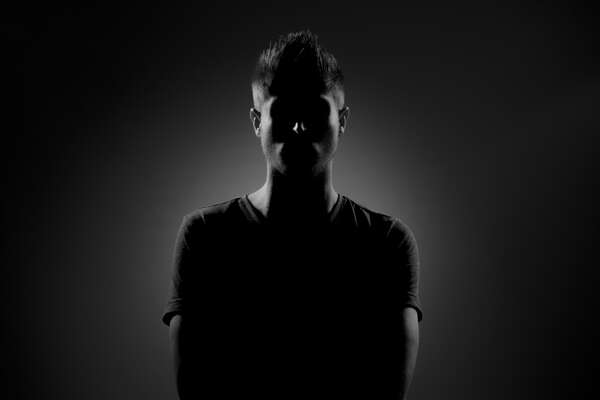
Anonymisation – Does John Doe exist?
The process of anonymising data is a hard and difficult task. How can you make sure that you have created John Doe – the dataset without personal content?
A train-the-trainer event on “Anonymisation for data sharing practices” took place on 4 October 2022. The main goal was twofold:
-
to show trainers the tools they need to teach the fundamentals of data anonymisation and disclosure control in training sessions
-
and to give them hands-on experience with current open source technologies (sdcMicro).
The training event was organised by the Croatian Social Science Data Archive (CROSSDA) and the Danish National Archives (DNA). The two archives presented how they managed the task of anonymisation.
Anonymisation at the Danish National Archives
When working with anonymisation at DNA, there are three main conditions that set the circumstances:
-
the Archives Act
-
the EU GDPR and
-
possible specific restrictions set by the donor.
Due to the Archives Act, DNA has the legal right to hold all kinds of personal data. And because Denmark is a country with a lot of registred data about its citizens, this means that DNA holds a lot of personal data.
At DNA a user can search here and all data, inclusive data with personal content will be presented with metadata explaining what the dataset holds. The data set can now be ordered in two versions: one with personal data and a version without – an anonymised version. If a researcher orders a data set with personal data, the researcher must have and show that they have the relevant permissions to access it.
Direct and indirect identifiers
A data set can contain lots of different personal information. When anonymising, the following data will always be removed:
-
civil registration number
-
name
-
email/phone number/address.
These are direct identifiers and therefore always removed.
Indirect identifies can be removed depending on individual cases and the circumstances around the data collection
Indirect identifiers are:
-
Job title
-
municipality
-
date and place of birth
-
nationality
-
religion
-
postal code
-
education
-
Other data revealed in free text.
Considerations to take when removing indirect identifiers
When deciding if these indirect identifiers must be removed the following conditions are taken into consideration.
The sensitivity of the research topic
If a research issue is sensitive, this will lead to stricter anonymisation and therefore more of the indirect identifiers will be removed. For example, sexual abuse, disease, sexual orientation, etc.
Specific interest
If the point of interest is very specific, this will automatically result in stricter anonymisation to make sure that a single individual cannot be identified.
The size and composition of the researched population
A small population will also lead to a more strict anonymisation. Otherwise, it would be easier to identify participants.
The presence of other information in data
Does the combination of info enable easy identification? This will be carefully considered.
The age of the data
The newer the data, the more strictly it will be anonymised, because it is likely to be more sensitive.
How does an archivist create John Doe?
A manual process starts when a user orders a data set with personal data but does not have the permission to acquire the full data set.
The process is done by an archivist who screens the data set for variables containing personal data and removes them. The screening and removal is then checked by a colleague. This additional step minimizes the risk of error.
During this second phase, edge cases can be identified. An edge case can be data sets where it is not possible to identify individuals, but when you hold other datasets and compare/or transfer data, there is a theoretical possibility. When both of these steps have been completed, DNA can send a fully anonymised dataset to the user. DNA has thus made sure that it is not possible to identify an individual.
SdcMicro – an open source tool
The process of anonymisation can be aided by the use of software, which is why the Croatian Social Science Data Archive (CROSSDA) presented SdcMicro, an open source R-package for generating protected microdata for researchers and public use.
A simple data set was prepared and used during the webinar to guide participants through the most important and basic functionalities of the tool. Attendees were given hands-on exercises to complete after the webinar and the test data set was shared as part of the train-the-trainer materials.
Some of the concepts and techniques that were presented included k-anonymity, top/bottom coding and recoding with practical examples and recommendations on how to incorporate anonymisation into research designs. Additionally, all of the methods were presented using the graphical user interface (sdcMictro GUI) to make the training more accessible to beginners.
K-anonymity is a property of a dataset that is designed to prevent a data subject from being singled out by grouping them with, at least k other individuals. This helps to ensure that an individual cannot be singled out based on their specific attribute values. In order to achieve k-anonymity, the attribute values of the individuals in the group must be generalised to some degree.
SdcMicro offers several methods for achieving k-anonymity, including top/bottom coding, also known as 'clipping'. This method censors data points whose values are outside of a certain range, typically by replacing them with a maximum or minimum value. Another way of doing this is by using global recoding, which involves replacing the original values of an attribute with a new set of values that are less specific. By using these and other techniques, it is possible to protect the privacy of individuals while still allowing for the use of data for research or other purposes.
Looking ahead
Creating John Doe – or anonymising a dataset to make sure that no individual can be traced – is a demanding task. In the case of the Danish National Archives, the process is manual. This is because their data can contain a lot of personal data. This also requires a case-by-case approach, based on several conditions as explained above.
Similar to DNA, CROSSDA also takes a case-by-case approach, and uses open source software to enhance the effectiveness of this process. Although still a young archive, CROSSDA is improving its methods and using community-made materials has been a great help.
If you want to create John Doe – the dataset without personal content, why not watch the recording and pay close attention to the process explained by the Danish National Archives?
The most important thing to remember is that there are no quick-fixes when it comes to anonymisation, because personal content can come in many shapes and forms.
More information:
-
Slides and materials are available on Zenodo https://doi.org/10.5281/zenodo.7139099
-
SdcMicro – an R-package to anonymise microdata
-
Further reading:
-
Anonymisation and Personal Data (Finnish Social Science Data Archive)
-
Anonymisation Decision Making Framework (UK Anonymisation Network)